Using what they call ‘game-powered machine learning’, the team says music lovers would be able to search every song on the web with a simple text search using key words like ‘funky’ or ‘spooky electronica’.
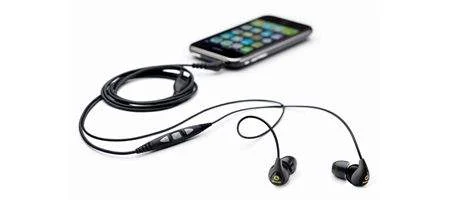
Searching for specific multimedia content, including music, isn’t easy because of the need to use text to search. There’s also the sheer volume of content to deal with: for example, YouTube users upload 60 hours of video content per minute.
Pandora, which relies on paid music experts, has just 900,000 songs in its catalog after 12 years in operation.
But the UC team says it’s possible to create a text-based multimedia search engine that will make it far easier to access multimedia content online.
Information on different types of music is gathered via a Facebook app called Herd It. Computers study examples of music that have been provided by music fans and labeled in categories such as ‘romantic’, ‘jazz’, ‘saxophone’ or ‘happy’ and then analyze them for acoustic patterns common to each pattern. It can then automatically label millions of songs by recognizing these patterns, says the team.
“This is a very promising mechanism to address large-scale music search in the future,” says electrical engineering professor Gert Lanckriet.
The machine can use what it has learned to ask for more information. For example, it might be great at recognizing the music patterns in rock music but struggle with jazz, in which case it might ask for more examples to study.
Through the active feedback loop, the computer automatically creates new Herd It games to collect the specific human input it needs to most effectively improve the auto-tagging algorithms, said Lanckriet.
Lanckriet foresees a time when – thanks to this massive database of cataloged music – phones will be able to provide a personalized radio service, matching music to one’s activity and mood, without repeating the same songs over and over again.
“What I would like long-term is just one single radio station that starts in the morning and it adapts to you throughout the day,” he says.
“By that I mean the user doesn’t have to tell the system, ‘Hey, it’s afternoon now, I prefer to listen to hip hop in the afternoon’. The system knows because it has learned the cell phone user’s preferences.”